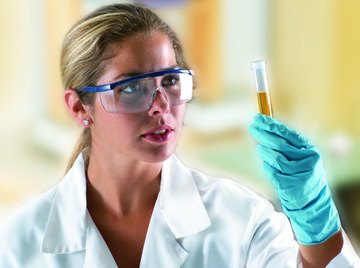
Sampling errors are the seemingly random differences between the characteristics of a sample population and those of the general population. For example, a study of the attendance at a monthly meeting reveals an average rate of 70 percent. Attendance at some meetings would certainly be lower for some than for others. The sampling error then is that while you can count how many people attended each meeting, what actually happens in terms of attendance at one meeting is not the same as what happens at the next meeting, even though the underlying rules or probabilities are the same. The keys to minimizing sampling error are multiple observations and larger samples.
Minimize the potential for bias in the selection of the sample through random sampling. Random sampling is not haphazard sampling but instead is a systematic approach to selecting a sample. For example, a random sample of a population of young offenders is generated by selecting names from a list to interview. Prior to seeing the list, the researcher identifies that young offenders to be interviewed as those whose names appear first, 10th, 20th, 30th, 40th and so on, on the list.
Ensure the sample is representative of the population by implementing a stratification protocol. For example, if you studied the drinking habits of university students, you might expect differences between fraternity students and non-fraternity students. Splitting your sample into those two strata at the outset reduces the potential for sampling error.
Use larger sample sizes. As the size increases, the sample gets closer to the actual population, thereby decreasing the potential for deviations from the actual population. For example, the average of a sample of 10 varies more than the average of a sample of 100. Larger samples do, however, involve higher costs.
Replicate your study by taking the same measurement repeatedly, using more than one subject or multiple groups, or by undertaking multiple studies. Replication allows you to swamp out sampling errors.
References
- University of Texas; Chapter 10. Data-Why Error is Unavoidable"; Craig M. Pease et. al.
- University of Texas; Chapter 11. Reducing the Error-A Template for Ideal Data; Craig M. Pease et. al.
- University of California Museum of Paleontology: Sampling Error and Evolution
- "Criminal Justice Research Methods Qualitative and Quantitative Approaches"; W. Lawrence Neuman et. al.; 2004
About the Author
Carol Strider is a writer and a post-secondary educator in law and criminal justice, teaching in person and online since 2002. Prior to teaching, Strider was a lawyer at a community law office. Strider holds a Juris Doctor, a Bachelor of Arts, a diploma in adult education and a diploma in animal sciences.
Photo Credits
Photos.com/liquidlibrary/Getty Images