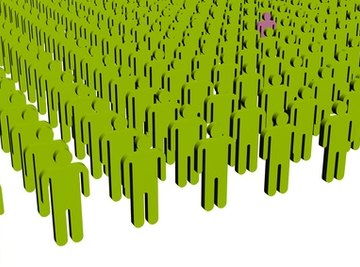
Sample size represents the number of observations taken to conduct a statistical analysis. Sample sizes can be composed of people, animals, food batches, machines, batteries or whatever population is being evaluated.
Random Sampling
Random sampling is a method by which random samples are collected from a population in order to estimate information about the population without being biased. For example, if you want to know what type of people live in a certain town, you have to interview/measure different people at random. However, if you just used everybody from the library, you would not have a fair/unbiased estimate of what the general population who occupy the town are like, just the people who go to the library.
Precision
As sample sizes increase, estimates become more accurate. For example, if we randomly selected 10 male adult humans, we might find their average height to be 6-feet-3-inches tall, perhaps because there is a basketball player that inflates our estimate. If, however, we measured two million adult male humans, we would have a better predictor of the mean height of males because the extremes would balance out and the true average would overshadow any deviations from the mean.
Confidence Intervals
When a statistician makes a prediction about an outcome, he will often build an interval around his estimate. For example, if we measured the weight of 100 women, we could say we are 90 percent confident that the true, average weight of women is in the interval of 103 to 129 pounds. (This, of course, depends on other factors like variability in the measurements as well.) As sample size increases, we become more confident about our estimate, and our intervals become smaller. For instance, with a million women, we could say we are 98 percent confident that the true, average weight of women is between 115 and 117 pounds. In other words, as sample size increases, our confidence in our measurements increases and the size of our confidence intervals decreases.
Standard Error
Variation is a measure of the spread of data around the mean. Standard deviation is the square root of variation and helps approximate what percentage of the population falls between a range of values relative to the mean. As the sample size increases, standard error, which depends on standard deviation and sample size, decreases. Consequently, estimates increase in precision and research built on this estimates is considered more reliable (with less risk of error).
Difficulty in Using Larger Sample Sizes
Larger sample sizes obviously produce better, more accurate estimates about populations, but there are several problems with researchers using larger sample sizes. First of all, it may be hard to find a random sample of people willing to try a new drug. When you do, it becomes costlier to provide the drug to more people and to monitor more people over time. Additionally, it takes more effort to gain and maintain a larger sample size. Even if larger sample sizes produce more accurate statistics, the extra cost and effort is not always needed as smaller sample sizes can also produce significant results.
References
- "Sample Sizes for Clinical Trials"; Steven Julious; 2009
About the Author
Sky Smith has been writing on psychology, electronics, health and fitness since 2002 for various online publications. He graduated from the University of Florida with honors in 2005, earning a Bachelor of Science in psychology and statistics with a minor in math.
Photo Credits
odd one out image by Daniel Wiedemann from Fotolia.com